Predicting beta bursts from local field potentials to improve closed-loop DBS paradigms in Parkinson’s patients
The severity of motor symptoms of Parkinson's disease is related to the appearance of prolonged periods of brain rhythms (or 'oscillations') in a particular frequency range, known as beta. These longer beta oscillations are disturbed by adaptive Deep Brain Stimulation, a promising therapy for Parkinson's. This paper investigated the ability of machine learning algorithms to predict the start of these beta oscillations. Such predictions would be useful for the control of adaptive Deep Brain Stimulation, namely for turning it on before the bursts start.
Motor symptoms in Parkinson’s disease (PD) correlate with an excess in synchrony in the beta frequency band (13-30Hz) of local field potentials recorded from basal ganglia circuits. Recent results have suggested that this abnormal activity arises as a result of changes in specific dynamical features of the underlying neural signatures. In particular, patterns of activity in the beta band have been shown to be structured in bursts of longer durations and higher amplitudes in untreated patients with PD. Closed-loop deep brain stimulation (DBS) paradigms that specifically target these pathological bursts of activity hold promises to help trim, and thus normalize, their abnormal behavior in real-time. Here, we developed classification algorithms that predict pathological beta bursts based on ongoing changes in LFP frequency dynamics. We then compared simulations of prediction-based DBS profiles with existing ‘adaptive DBS’ alternatives. We show that model-driven stimulation profiles are more precise in restricting the delivery of stimulation to bursts that are considered pathological, while preserving physiological ones. The overall stimulation time required is also diminished, thus supporting longer battery life. These results represent a conceptual and algorithmic framework for the development of more precise DBS strategies that are selectively tailored to the electrophysiological profile of each patient.
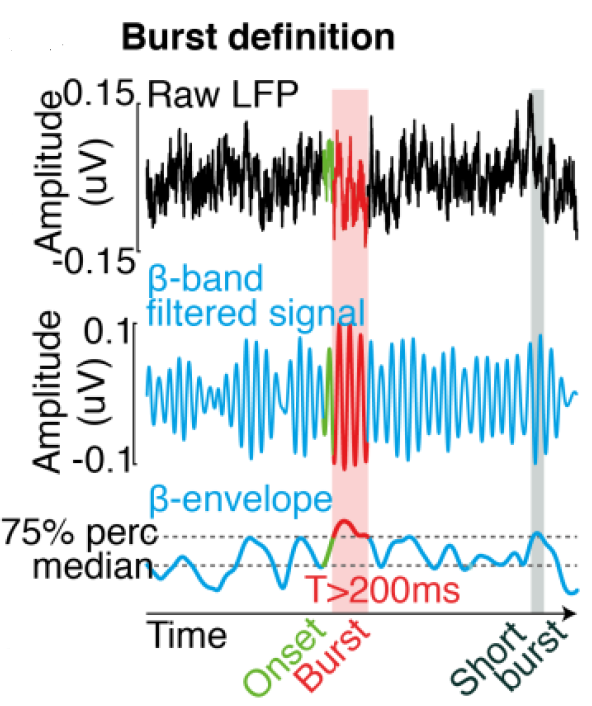
2021. Mov Disord, 36(4):863-873.
2024. Neurobiol Dis, 201:106652.