EMD: Empirical Mode Decomposition and Hilbert-Huang Spectral Analyses in Python
Scientific Abstract
The Empirical Mode Decomposition (EMD) package contains Python (>=3.5) functions for analysis of non-linear and non-stationary oscillatory time series. EMD implements a family of sifting algorithms, instantaneous frequency transformations, power spectrum construction and single-cycle feature analysis. These implementations are supported by online documentation containing a range of practical tutorials.
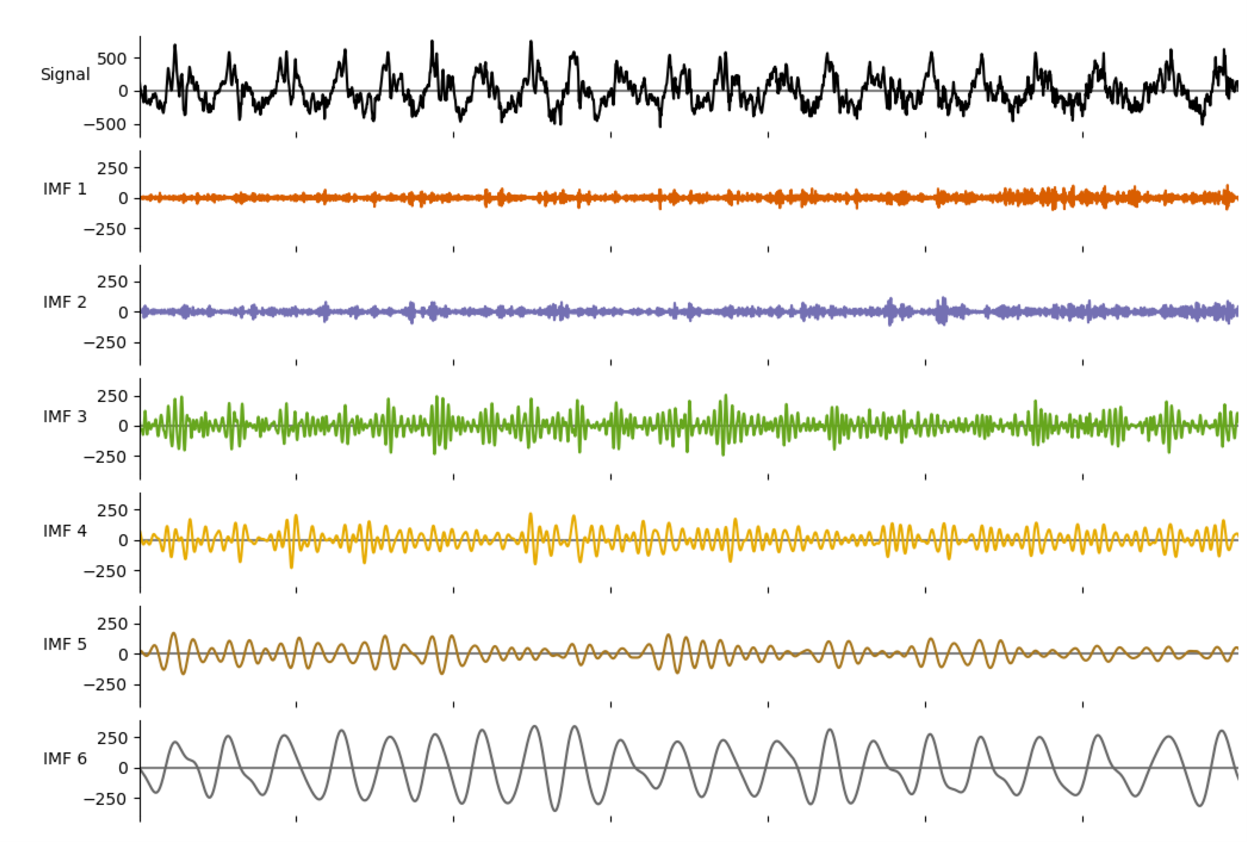
A segment of the LFP recording separated into six intrinsic mode functions using a masked empirical mode decomposition. The theta oscillation is isolated into IMF 6.
Citation
Journal of Open Source Software, 6(59), 2977.
DOI
10.21105/joss.02977
Related Content
Publication
2022. Exp Neurol, 351:113999.
Publication
2018. Neuron, 100(4):940–952.
Publication
2022. Brain, 145(1):237-250.
Publication
2023. Mov Disord, 38(5):818-830.
Publication
2017.Proc. Natl. Acad. Sci. U.S.A., 114(26):6666-6674.