Application of Machine Learning Using Decision Trees for Prognosis of Deep Brain Stimulation of Globus Pallidus Internus for Children With Dystonia.
Dystonia in childhood can significantly impact on quality of life. Some affected children benefit from a type of surgery called deep brain stimulation, but which ones? Here, we use a form of machine learning to define those clinical characteristics and tests that help predict improvement after surgery.
Background: While Deep Brain Stimulation (DBS) of the Globus pallidus internus is a well-established therapy for idiopathic/genetic dystonia, benefits for acquired dystonia are varied, ranging from modest improvement to deterioration. Predictive biomarkers to aid DBS prognosis for children are lacking, especially in acquired dystonias, such as dystonic Cerebral Palsy. We explored the potential role of machine learning techniques to identify parameters that could help predict DBS outcome.
Methods: We conducted a retrospective study of 244 children attending King’s College Hospital between September 2007 and June 2018 for neurophysiological tests as part of their assessment for possible DBS at Evelina London Children’s Hospital. For the 133 individuals who underwent DBS and had 1-year outcome data available, we assessed the potential predictive value of six patient parameters: sex, aetiology (including cerebral palsy), baseline severity (Burke-Fahn-Marsden Dystonia Rating Scale-motor score), cranial MRI and two neurophysiological tests, Central Motor Conduction Time (CMCT) and Somatosensory Evoked Potential (SEP). We applied machine learning analysis to determine the best combination of these features to aid DBS prognosis. We developed a classification algorithm based on Decision Trees (DTs) with k-fold cross validation for independent testing. We analysed all possible combinations of the six features and focused on acquired dystonias.
Results: Several trees resulted in better accuracy than the majority class classifier. However, the two features that consistently appeared in top 10 DTs were CMCT and baseline dystonia severity. A decision tree based on CMCT and baseline severity provided a range of sensitivity and specificity, depending on the threshold chosen for baseline dystonia severity. In situations where CMCT was not available, a DT using SEP alone provided better than the majority class classifier accuracy.
Conclusion: The results suggest that neurophysiological parameters can help predict DBS outcomes, and DTs provide a data-driven, highly interpretable decision support tool that lends itself to being used in clinical practice to help predict potential benefit of DBS in dystonic children. Our results encourage the introduction of neurophysiological parameters in assessment pathways, and data collection to facilitate multi-centre evaluation and validation of these potential predictive markers and of the illustrative decision support tools presented here
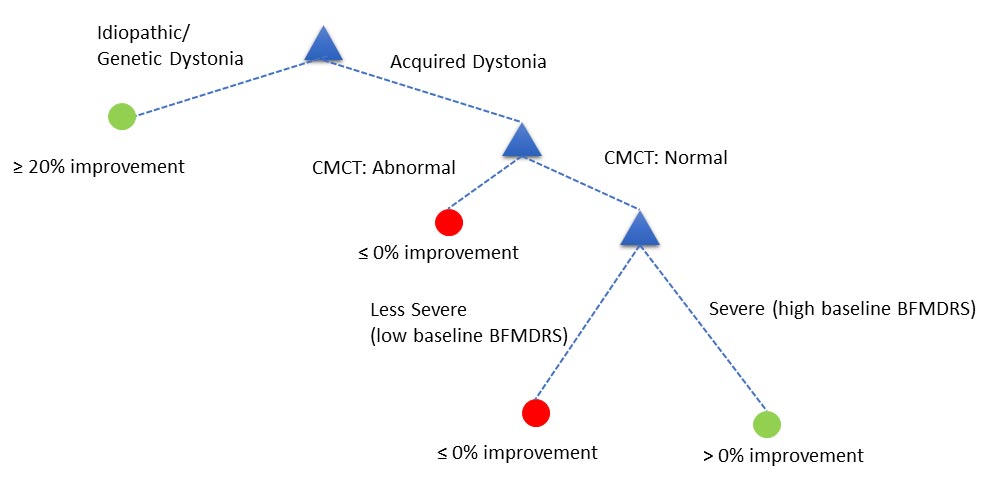
2023. Neuromodulation, 26(2):320-332.
2023. Mov Disord, 38(3):423-434.