Optimizing deep brain stimulation based on isostable amplitude in essential tremor patient models.
The size (‘amplitude’) of pathological brain activity can be quantified using the mathematical concept of ‘isostable amplitude’. Here, we show that using this definition of amplitude could help improve deep brain stimulation for patients with essential tremor.
Deep brain stimulation is a treatment for medically refractory essential tremor. To improve the therapy, closed-loop approaches are designed to deliver stimulation according to the system's state, which is constantly monitored by recording a pathological signal associated with symptoms (e.g. brain signal or limb tremor). Since the space of possible closed-loop stimulation strategies is vast and cannot be fully explored experimentally, how to stimulate according to the state should be informed by modeling. A typical modeling goal is to design a stimulation strategy that aims to maximally reduce the Hilbert amplitude of the pathological signal in order to minimize symptoms. Isostables provide a notion of amplitude related to convergence time to the attractor, which can be beneficial in model-based control problems. However, how isostable and Hilbert amplitudes compare when optimizing the amplitude response to stimulation in models constrained by data is unknown.
We formulate a simple closed-loop stimulation strategy based on models previously fitted to phase-locked deep brain stimulation data from essential tremor patients. We compare the performance of this strategy in suppressing oscillatory power when based on Hilbert amplitude and when based on isostable amplitude. We also compare performance to phase-locked stimulation and open-loop high-frequency stimulation.
For our closed-loop phase space stimulation strategy, stimulation based on isostable amplitude is significantly more effective than stimulation based on Hilbert amplitude when amplitude field computation time is limited to minutes. Performance is similar when there are no constraints, however constraints on computation time are expected in clinical applications. Even when computation time is limited to minutes, closed-loop phase space stimulation based on isostable amplitude is advantageous compared to phase-locked stimulation, and is more efficient than high-frequency stimulation.
Our results suggest a potential benefit to using isostable amplitude more broadly for model-based optimization of stimulation in neurological disorders.
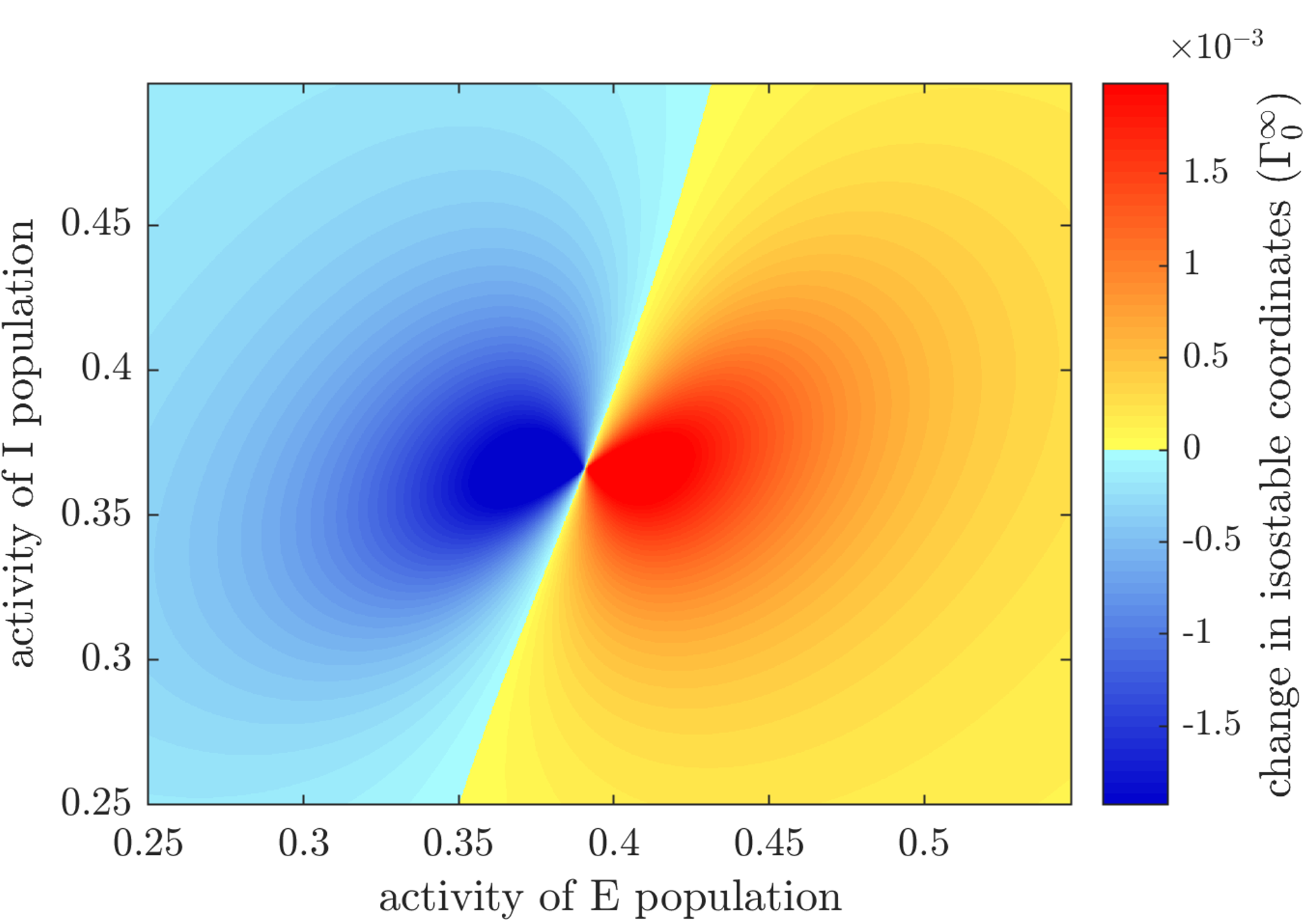
2021. J Neural Eng, 18(4):046023.
2021. Mov Disord, 36(4):863-873.
2025. Neurobiol Dis, 207:106858.