Learning Reward Uncertainty in the Basal Ganglia.
This paper describes the development and use of a mathematical model to explore how a group of brain regions called the basal ganglia can learn about the spread and average sizes of rewards associated with performing different actions. The model also proposes how brain nerve cells producing the chemical messenger dopamine can control how we avoid risky actions associated with variable rewards.
Learning the reliability of different sources of rewards is critical for making optimal choices. However, despite the existence of detailed theory describing how the expected reward is learned in the basal ganglia, it is not known how reward uncertainty is estimated in these circuits. This paper presents a class of models that encode both the mean reward and the spread of the rewards, the former in the difference between the synaptic weights of D1 and D2 neurons, and the latter in their sum. In the models, the tendency to seek (or avoid) options with variable reward can be controlled by increasing (or decreasing) the tonic level of dopamine. The models are consistent with the physiology of and synaptic plasticity in the basal ganglia, they explain the effects of dopaminergic manipulations on choices involving risks, and they make multiple experimental predictions.
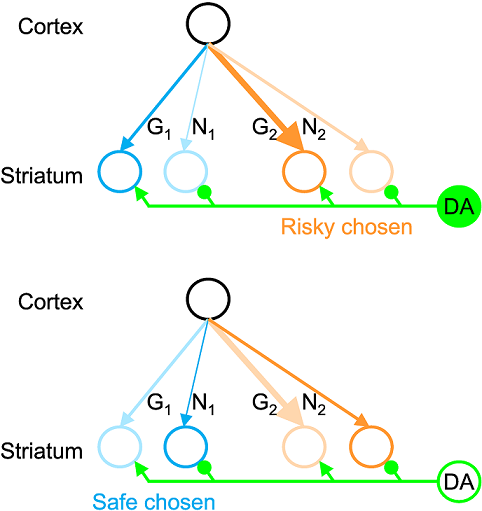
2016.PLoS Comput. Biol., 12(9):e1005062.
2022. PLoS Comput Biol, 18(5)e1009816.
2017.Neural Comput, 29(2):368-393.