Expressions for Bayesian confidence of drift diffusion observers in fluctuating stimuli tasks
Several theories have been proposed on how the brain computes confidence during decision making. However, some of the theories are difficult to compare with experimental data because the computer simulations of the models they assume take too long. This paper presents an efficient method for comparing predictions of models of confidence with the data.
We introduce a new approach to modelling decision confidence, with the aim of enabling computationally cheap predictions while taking into account, and thereby exploiting, trial-by-trial variability in stochastically fluctuating stimuli. Using the framework of the drift diffusion model of decision making, along with time-dependent thresholds and the idea of a Bayesian confidence readout, we derive expressions for the probability distribution over confidence reports. In line with current models of confidence, the derivations allow for the accumulation of “pipeline” evidence that has been received but not processed by the time of response, the effect of drift rate variability, and metacognitive noise. The expressions are valid for stimuli that change over the course of a trial with normally-distributed fluctuations in the evidence they provide. A number of approximations are made to arrive at the final expressions, and we test all approximations via simulation. The derived expressions contain only a small number of standard functions, and require evaluating only once per trial, making trial-by-trial modelling of confidence data in stochastically fluctuating stimuli tasks more feasible. We conclude by using the expressions to gain insight into the confidence of optimal observers, and empirically observed patterns.
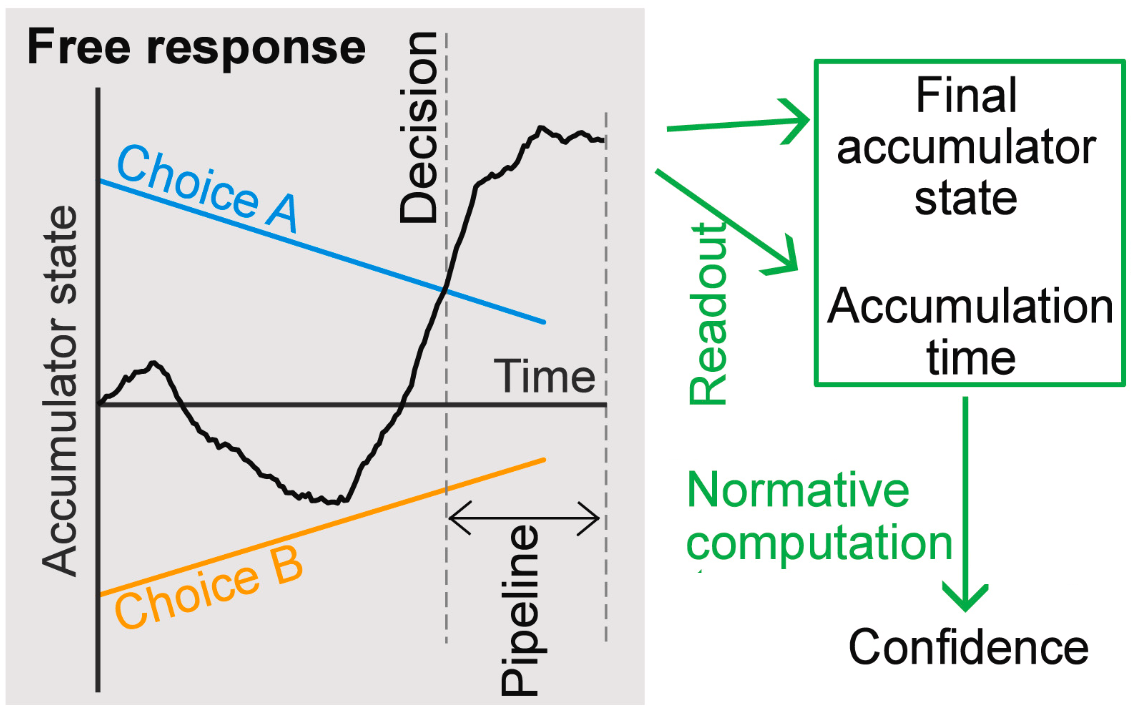