A deep learning framework for neuroscience.
In this paper, we discuss how neuroscience research could benefit from recent advances in artificial intelligence. New and sophisticated computer programs, known as artificial neural networks, can learn complex tasks like naming images, translating languages, or playing board games. We argue that the insights gained from studying artificial neural networks could provide a framework to investigate the mechanisms of learning in the real brain.
Systems neuroscience seeks explanations for how the brain implements a wide variety of perceptual, cognitive and motor tasks. Conversely, artificial intelligence attempts to design computational systems based on the tasks they will have to solve. In artificial neural networks, the three components specified by design are the objective functions, the learning rules and the architectures. With the growing success of deep learning, which utilizes brain-inspired architectures, these three designed components have increasingly become central to how we model, engineer and optimize complex artificial learning systems. Here we argue that a greater focus on these components would also benefit systems neuroscience. We give examples of how this optimization-based framework can drive theoretical and experimental progress in neuroscience. We contend that this principled perspective on systems neuroscience will help to generate more rapid progress.
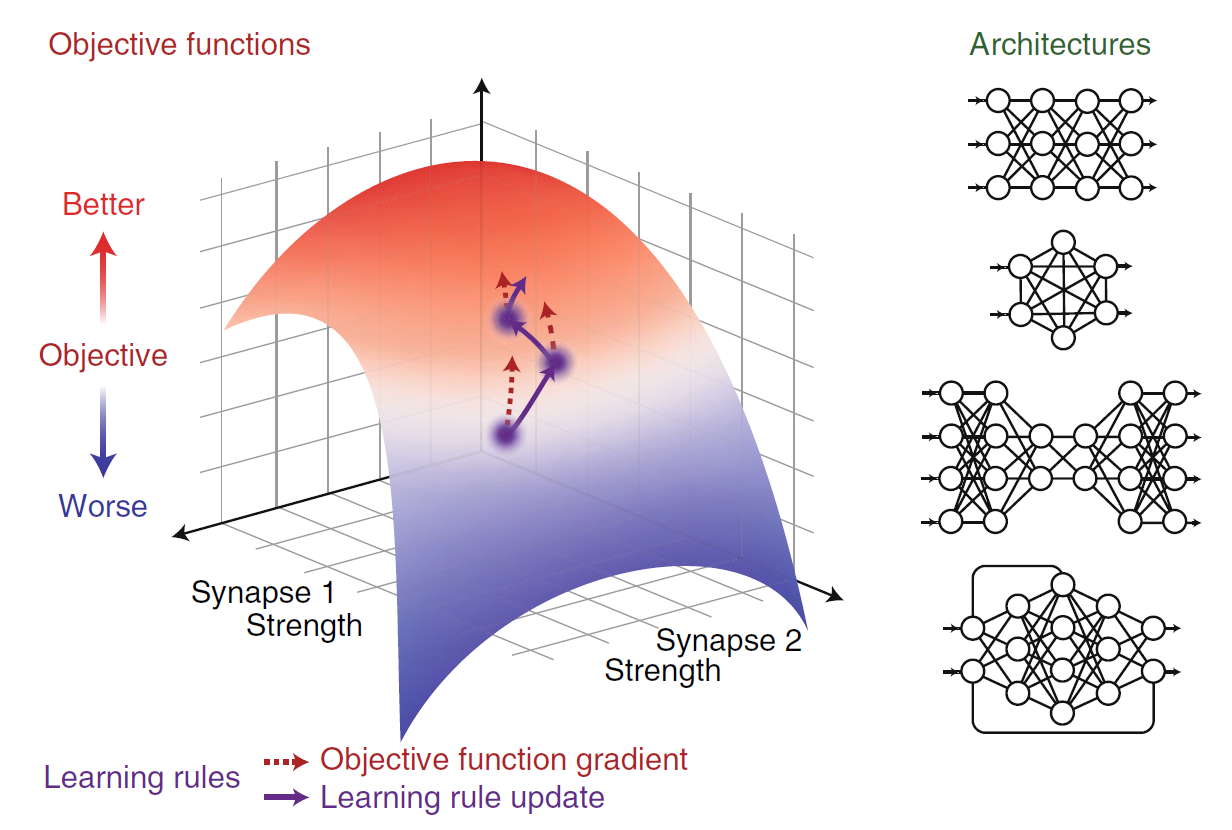