Sequential Memory with Temporal Predictive Coding
Memorizing and remembering things in order, like the sequence of turns on a path home, is something animals, including humans, do naturally. In our research, we've developed a computer model that tries to replicate how the brain might handle this task of remembering sequences by predicting what comes next. We call this approach 'temporal predictive coding'. This model imitates the way the brain learns and reacts to sequences, offering a new insight into how it manages to keep different sequence memories from getting mixed up.
Forming accurate memory of sequential stimuli is a fundamental function of biological agents. However, the computational mechanism underlying sequential memory in the brain remains unclear. Inspired by neuroscience theories and recent successes in applying predictive coding (PC) to \emph{static} memory tasks, in this work we propose a novel PC-based model for \emph{sequential} memory, called \emph{temporal predictive coding} (tPC). We show that our tPC models can memorize and retrieve sequential inputs accurately with a biologically plausible neural implementation. Importantly, our analytical study reveals that tPC can be viewed as a classical Asymmetric Hopfield Network (AHN) with an implicit statistical whitening process, which leads to more stable performance in sequential memory tasks of structured inputs. Moreover, we find that tPC exhibits properties consistent with behavioral observations and theories in neuroscience, thereby strengthening its biological relevance. Our work establishes a possible computational mechanism underlying sequential memory in the brain that can also be theoretically interpreted using existing memory model frameworks.
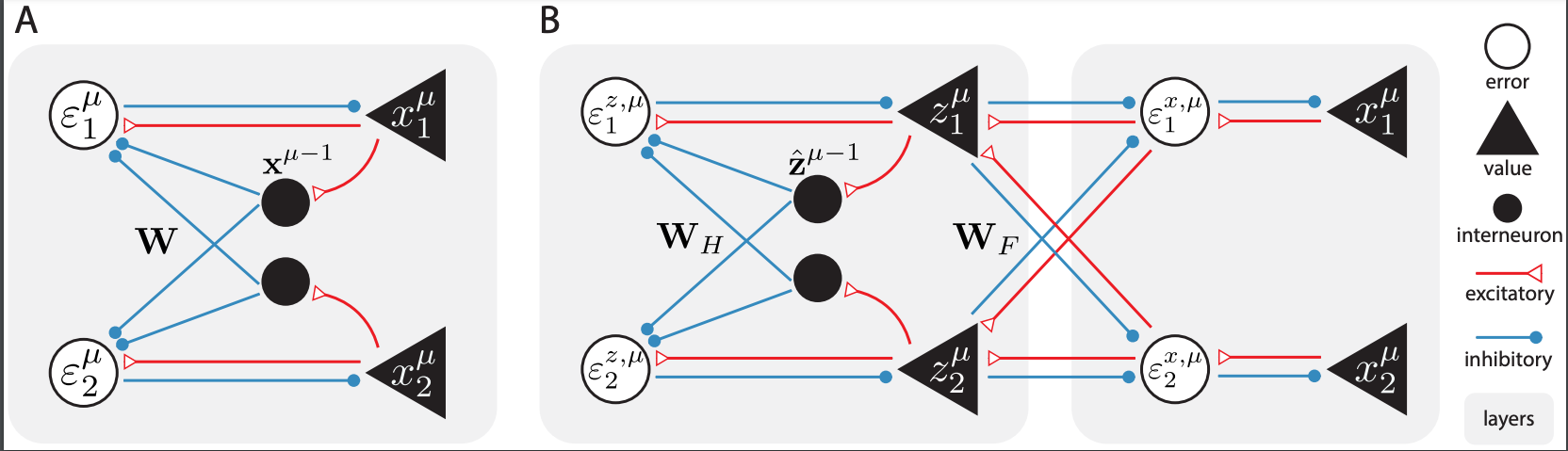
2023. PLoS Comput Biol, 19(4)e1010719.